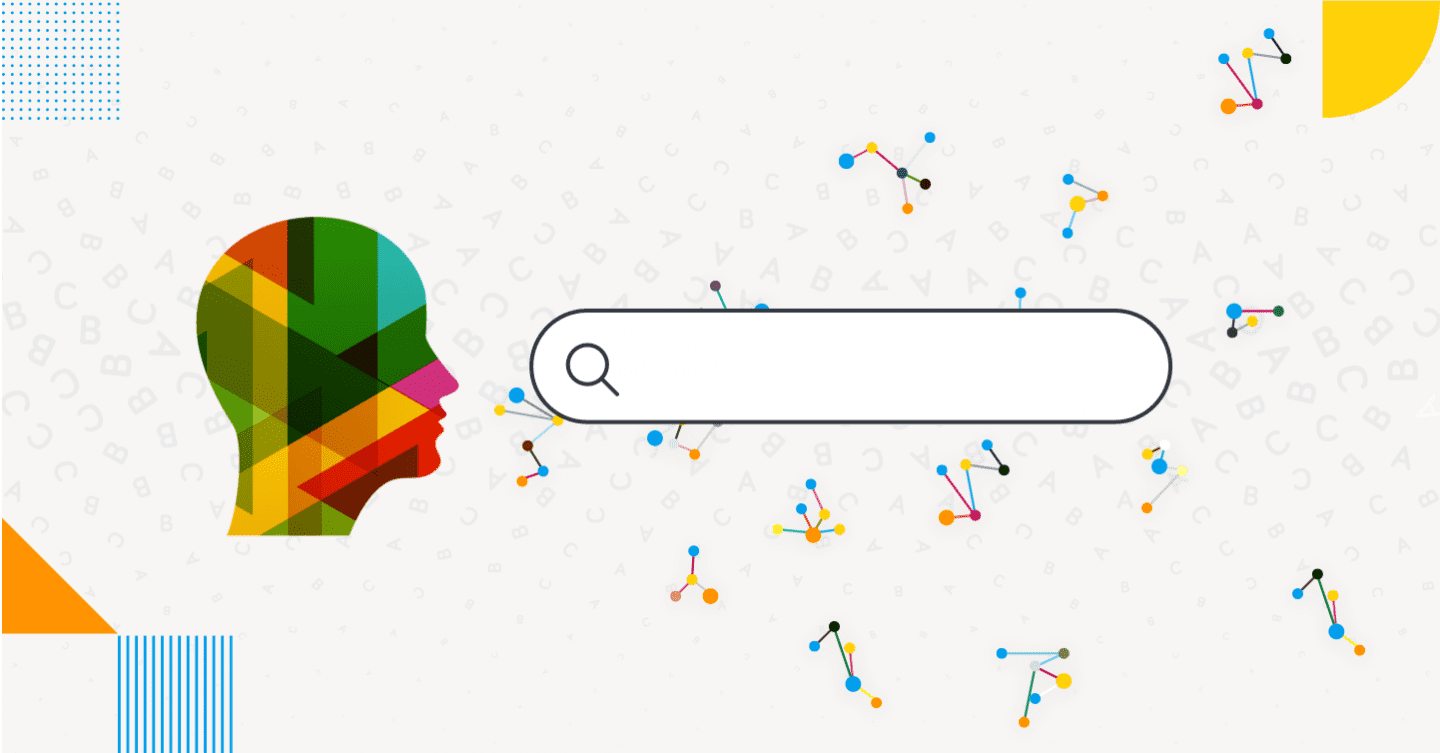
What is natural language query (NLQ)?
Providing your analytics users the ability to get answers from their data is useful, but only if the solution can guide them to ask the right questions.
The rising integration of artificial intelligence (AI) and machine learning (ML) in many business intelligence (BI) solutions has enabled new innovative approaches in combining natural language techniques with self-service analytics, resulting in helpful NLQ tools.
But what is natural language query, exactly, and why is it now important? Is it the same thing as typing in a question in a search engine, or something more? And how is it different from other natural language techniques out there? This article aims to explain its many nuances, and the unique NLQ solutions currently available from BI vendors.
What is natural language query (NLQ)?
Natural language query (NLQ) is a capability in BI software solutions that allows people to ask questions of data within their analytics platform, using everyday language as they would to another person, to find information they need to make business decisions.
Depending on the sophistication of the NLQ offering, analytics users can query data using terms either typed, or spoken into a search box. The BI system then parses for keywords, searches relevant databases and generates an answer, typically using a report or chart that attempts to answer the query, and ideally helps provide understanding.
NLQ tools come in different forms and levels of integration, varying between software vendors. Some platforms incorporate voice interaction and queries through a virtual personal assistant, and support integration on mobile phones and other smart devices. NLQ can also be combined with conversational AI solutions, such as virtual artificial intelligence (AI) assistants, or chatbots.
NLQ sits under an umbrella of natural language technologies that enable new ways to not just communicate information between humans and systems, but explain data and analysis in a way that is accessible to those not as analytically inclined. The other fields of natural language commonly used in analytics include natural language processing (NLP), natural language generation (NLG) and natural language understanding (NLU).
What is the purpose of using NLQ for BI?
The basis of natural language query would be familiar to anyone who has used Google.
You type in a set of keywords, and the system instantly matches them with relevant information found in related databases, providing a list of results with a set of answers.
In analytics, however, its core purpose is different.
Modern BI solutions combine NLQ with embedded AI and machine learning models, generated from augmented analytics, for the purpose of assisting non-technical users to analyze their data easier and faster, as part of the rise of the augmented consumer trend.
NLQ is another effective way to democratize self-service analytics in the business, and make more information accessible to more people, not just data experts or analysts, who are searching for it, which is why it's now much more available. In short, NLQ can help:
- Assist non-technical people by providing another way to explore their data
- Enhance other traditional analysis techniques, such as report building
- Make the use of analytics more pervasive throughout the organization overall
With NLQ, instead of having to manually dig into data, an analytics user can theoretically get started on their journey for answers by asking questions of the system directly, and get a relevant answer, rather than having to rely solely on finding answers in a traditional dashboard or chart - which not all non-technical BI users would be able to do.
NLQ’s overall rise in prominence comes as analytics vendors have begun to offer other modern tools to streamline the analysis process for all users, not just experts and analysts, including data storytelling and automated business monitoring.
What does NLQ offer for software companies and enterprise analytics users?
Self service analytics need to match its users. Even though many software vendors have made BI easier with better user interfaces and streamlined processes, it still takes a lot of technical knowledge, data literacy and skill to simply use many of these tools.
For example, your Sales team may never build a report or chart just because some self-service dashboard tools are available. They may not have the time, skills, or the time to pick up the necessary knowledge. These options better suit data analysts or reasonably technical dashboard creators, who make complex reports daily.
NLQ can help change that, for non-technical users who might not be as well-versed with traditional BI solutions (i.e. don’t have the time), or who simply don’t get the same level of understanding others may from consuming a dashboard or a chart.
For Independent Software Vendors (ISVs): With NLQ available, software companies can further open up true self-service analytics for their customers without adding to the support burden of IT, by enabling the system to provide answers to data queries. As it stands today, the ISV has to help customize many reports for their customers or field consulting services to do so.
For Enterprise: NLQ can help increase adoption of analytics platforms because more users will be able to use it, in particular much-neglected, non-technical business users.
The growing pains of NLQ analytics - and its future
The capability for software systems to parse structured data and generate text and spoken language from it is far from a new technology or technique. Rather, the gradually increasing sophistication in its ability to generate truly relevant answers, has been.
But, as we’ve covered previously, many NLQ solutions, (also called search-based BI tools) available in the market today have not delivered on expectations and promises, or failed to provide an effective solution for non-technical users to get answers, resulting in wasted potential.
Thankfully, a new approach to NLQ arrived with the release of Guided NLQ, Yellowfin's exclusive natural language query feature that allows your users to type a question about their data and gain instant, automated recommendations and parameters in a drop-down menu that ensure they structure their query in the correct way, and receive relevant answers in the form of generated best practice data visualizations and dashboards.
At Yellowfin, we believe in a different approach to leveraging the possibilities that NLQ can unlock. While everyone else is focusing on providing a search-based experience for their users, we see our Guided NLQ feature, and NLQ as a technology, working best as a guided experience. And this radically different approach is the way to go, to ensure self-service analytics is democratized for every user. To learn more about Guided NLQ and how Yellowfin is changing the game with augmented, AI-powered analytics features, download our free whitepaper, Embedding AI-Powered Analytics Into Your Application.
The future of NLQ is guided, not search-based
Learn why traditional search-based NLQ just hasn't lived up to expectations, and how a new guided approach to NLQ will open up analytics for everyone with Yellowfin's Guided NLQ feature.