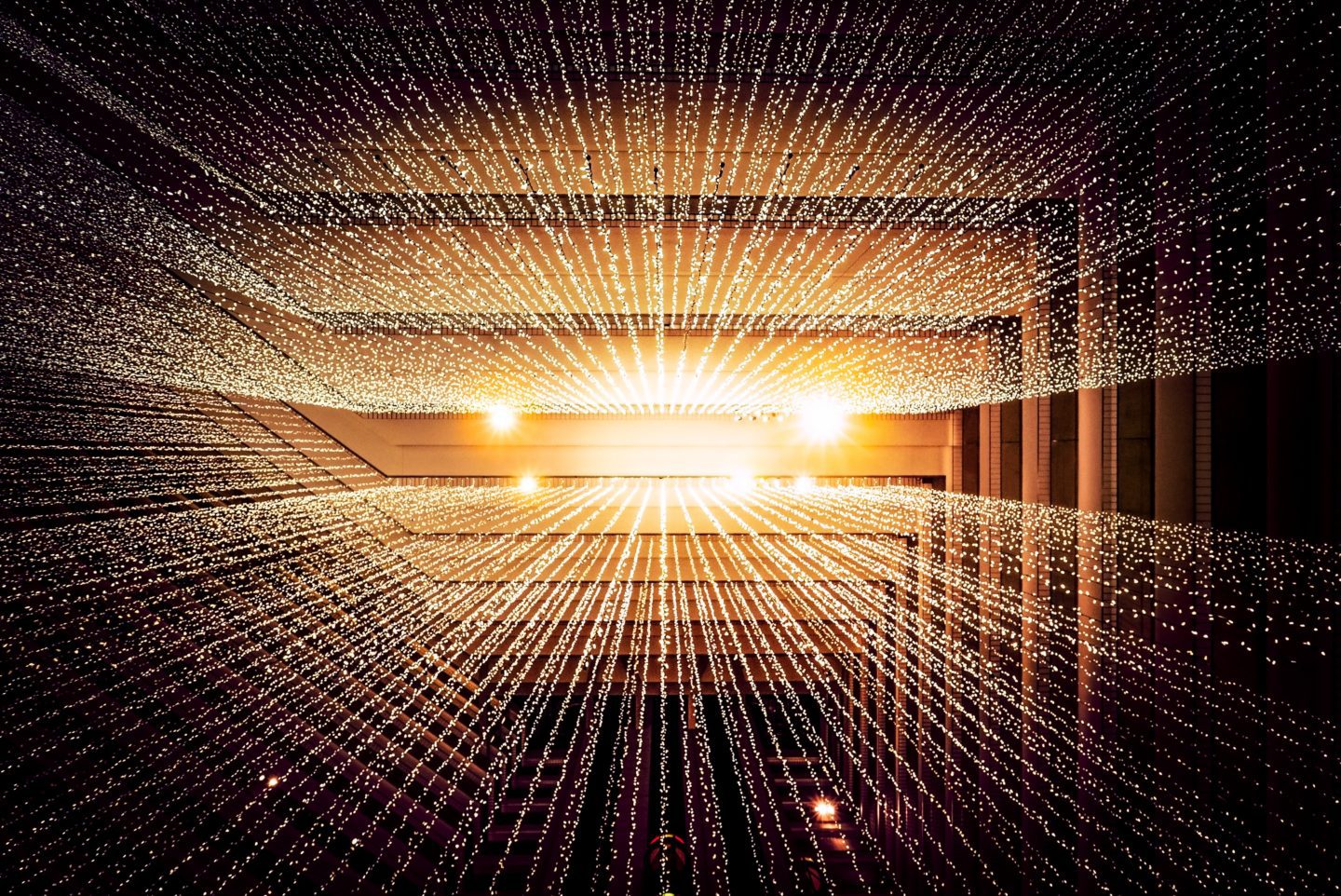
Everything we didn't know about data discovery until we started trying to automate it
In Yellowfin’s 7.4 release, we introduced an automated data discovery module. When we started developing this module we didn’t have an honest understanding of the many challenges analysts face using existing tools for data discovery. It quickly became apparent that the BI industry had been pushing the workload of discovery into analyst driven data manipulation. BI platforms didn’t take data, process it, and ask questions without a substantial amount of massaging from a data analyst.
Once we had our light bulb moment we were able to see data discovery in a whole new light. It’s given us a radical departure from the industry norm and how other vendors think about data discovery and processing.
Ask users what they need, don’t assume
As we understood more about the challenges of data discovery, it quickly became apparent that we had been operating with two large blind spots.
The first blind spot was an industry-wide one. We looked at our functionality, compared it to our competitors and assumed we’d covered everything. We hadn’t considered that there was some essential functionality that didn’t exist in any product in the market, like automated data discovery.
The second blind spot was the assumptions that we made around how people used BI products. As we automated data discovery, we needed to understand exactly what questions a data scientist would ask of their data. So over the course of years, we spoke to many data scientists and found out what questions they asked as they prepared data for analysis. It sounds odd, but as we worked through this process I became very confident that not many other vendors have ever asked these questions.
Traditionally, it seems like many vendors haven’t really thought about the questions that people are asking of their data. But this is an essential step when you’re building an interface that allows people to easily ask their questions and return an answer immediately. A vendor’s role isn’t to assume what these questions are, it’s to automate them.
As our blind spots became apparent, the process of automation also became harder. While our initial spec was completed quickly, as we started to peel back the layers we discovered more things that we hadn’t really considered or thought of previously. This made it all the more exciting as we started to put all the pieces together.
The nuances in data discovery
Data discovery has traditionally been a completely manual process. Analysts have worked through data, slicing and dicing it manually. As a result, it’s easy to miss a lot of the nuances in the way data discovery should happen.
Take comparative analysis, for example. Comparative analysis is an important aspect of any data analyst’s work- comparing quarterly financials, one region to another, budget to actual, the use cases go on. To do this well it’s necessary to take the raw data and create sets. These sets then need to be filtered and the coefficient of variation across a range of data needs to be determined.
The work required to conduct comprehensive comparative analysis is almost impossible to do manually, yet data analysts were spending an inordinate amount of time trying every possible combination to find that nugget of gold. The BI tool should give this information to the analyst, yet only one platform that I’m aware of did this – until now. By automating comparative analysis, the data analyst no longer needs to waste time conducting comparative analysis by hand.
Profitability metrics are another example. While it’s relatively simple to work out the metrics manually, the comparative analysis required to gain insight into the data is more complex. By investigating this we were able to highlight the gaps in our existing product, like identifying outliers.
For example, if air travel costs have increased significantly from one quarter to another it might be easy to identify some significant outliers – perhaps a couple of people flew first class. But if the outliers are not so obvious – perhaps the price of one particular flight path has changed dramatically – it can be harder to find in the data manually. An analyst may previously have spent days slicing and dicing data trying to find this outlier, but the real value is in finding this information quickly, understanding it and then delivering that information back to the business.
These nuances are not easy to detect manually, but it wasn’t until we automated the process of data discovery that we could see how many more insights our customers could identify.
In the past year, we’ve transformed how people discover data. We’ve achieved this by breaking free from industry assumptions and norms and getting to the heart of how people use our product. This has allowed us to make it easier for users to conduct their analysis. Users can get on with the task of providing insights and value to their business.
Join a group demo to see how automated data discovery can change how your team discovers data.