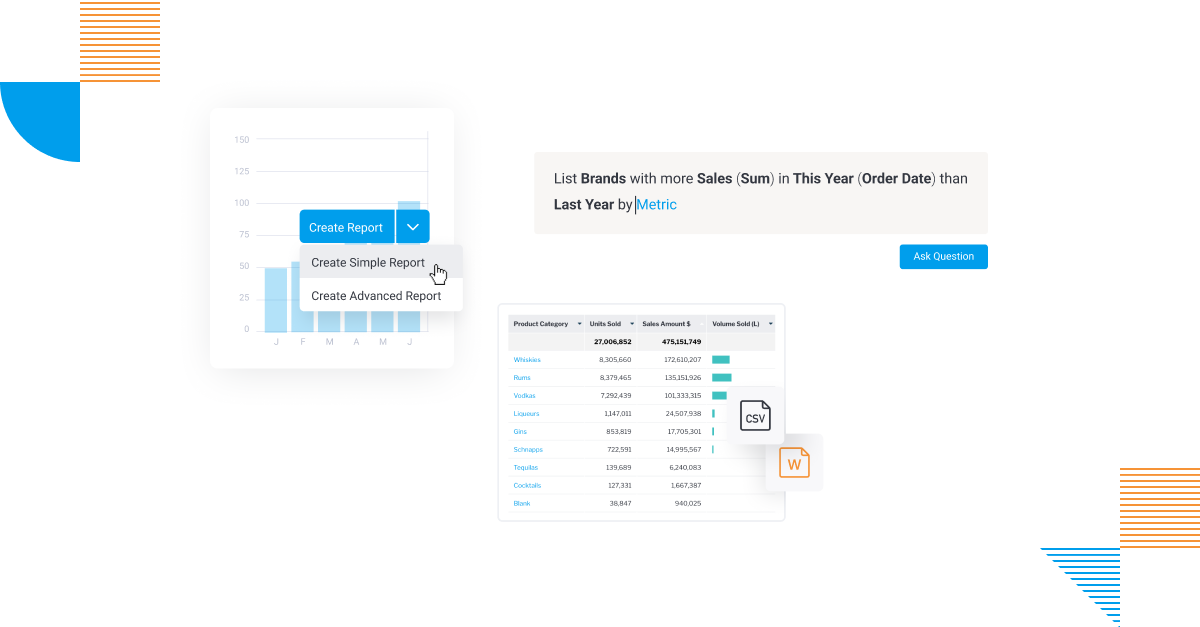
Traditional BI vs Self-Service Analytics: What's the Difference?
Data has historically been in the hands of a select few in most businesses - until recently.
Business intelligence (BI) solutions have evolved dramatically in the last few years to not only be more sophisticated, but simpler and more accessible for regular professionals to use analytics tools and get the insights they need to make decisions.
Instead of having to go to the IT department everytime a report needs to be requested, many modern BI solutions now allow users of all skill levels to explore and analyze their data through streamlined user experiences.
Two types of approaches to analytics have since emerged: Traditional, IT-managed BI, and a self-service BI model that puts the power of analytics in the hands of each user.
So, what are the differences between these two approaches, and which is better? The answer depends on your business case - but it helps to understand the differences first.
What is the Traditional BI Approach?
Traditional business intelligence describes a model of BI managed by an organization’s IT department or in-house data experts. The process of reporting, data preparation, storage and analysis, and insight sharing is entirely controlled by a select few.
This means in a traditional BI approach, line-of-business users are required to go to their IT department or in-house analyst any time they need to access important data, generate dashboards and reports, run queries or explain insights for their decision-making needs.
The reporting style of traditional BI is typically defined as parameter-driven dashboards and reports that provide granular data for a low-level view of the user’s most immediate areas of need (rather than a “big picture” view), helping users to act faster in the short-term. Reports and dashboard must first be requested by the user, then approved and prepared by IT, before they are built and shared with whomever needs the data. In essence, the analytic workflow, including analysis, is dependent on the technical department, rather than the user seeking insights.
Traditional BI is often used for business cases when daily operational reporting is a major requirement, such as for regulatory compliance reporting or weekly sales leads tracking. It’s also helpful for when an organization wants to tightly control the datasets available to their users, to ensure they are only consuming and sharing accurate, relevant data vetted by the organization.
How Did Traditional BI Start?
Analysts, data scientists, and IT have typically been the only people in most businesses that are able to use BI solutions, on account of the inherent complexity of most traditional analytics tools.
With the need for specific expertise to be able to extract, transform and load (ETL) data into a data warehouse, or write SQL queries to generate reports before any data can even be analyzed, a lot of training was additionally required from non-experts in order to begin analyzing and reporting on data. Much of this process is now automated today, but legacy tools often lacked this level of streamlining, forcing IT departments or technical developers to manage it.
Traditionally, most BI tools have been built to be used by more technically-inclined users, despite the fact that line-of-business users could benefit most from visual-based exploration of their data using dashboards, data visualization, and data stories.
Slowly, this has begun to change as BI vendors increasingly streamline their user experience with drag-and-drop interfaces, easy to use design dashboard canvases and automated one-click analysis features to open up their suite for more people, but centralized analytics remains prominent among enterprise and SMB today.
Read More: The History and Evolution of Business Intelligence (BI) Platforms
What are the Pros and Cons of Traditional BI?
Traditional, IT-managed BI brings certain advantages, but also some limitations.
With tight control over data and the right IT department and analysts managing it, it can be easier to ensure strict data security and data governance practices are enforced, as well as the design, format, quality and accuracy of the data which users will request detailed answers from.
Traditional analytics also allows organizations to offer a scalable and standardized reporting process for its users to reliably source data-backed answers to their business questions, typically functional overviews on “what has happened in the past?” and “what is happening now?”
Where it may not be appropriate is if your organization’s IT resources are stretched thin. Business data is continuously growing, so if your IT department struggles to keep up with data preparation or can’t deliver users their requested reports or queries in a timely manner, or your line-of-business users demand more accessible tools to be able to proactively explore data themselves, it may be time for your organization to investigate new BI options.
Otherwise, users may start making decisions without data, or use other non-vetted analytics tools.
If you find your users are demanding more capability to find answers to their spur of the moment questions on their own, it’s a good sign your user base has outgrown the IT centralized model of BI.
IT centralized analytics also creates potential problems for your technical departments. If your IT department has to constantly answer requests from users for reports and dashboards, it may mean they have less time to focus on other important areas of the business.
Such analytics was once the only approach to BI, until software vendors began to build and offer analytics solutions that are not only more accessible to line-of-business users, but tailored around their needs. This new approach is called self-service analytics, or self-service BI.
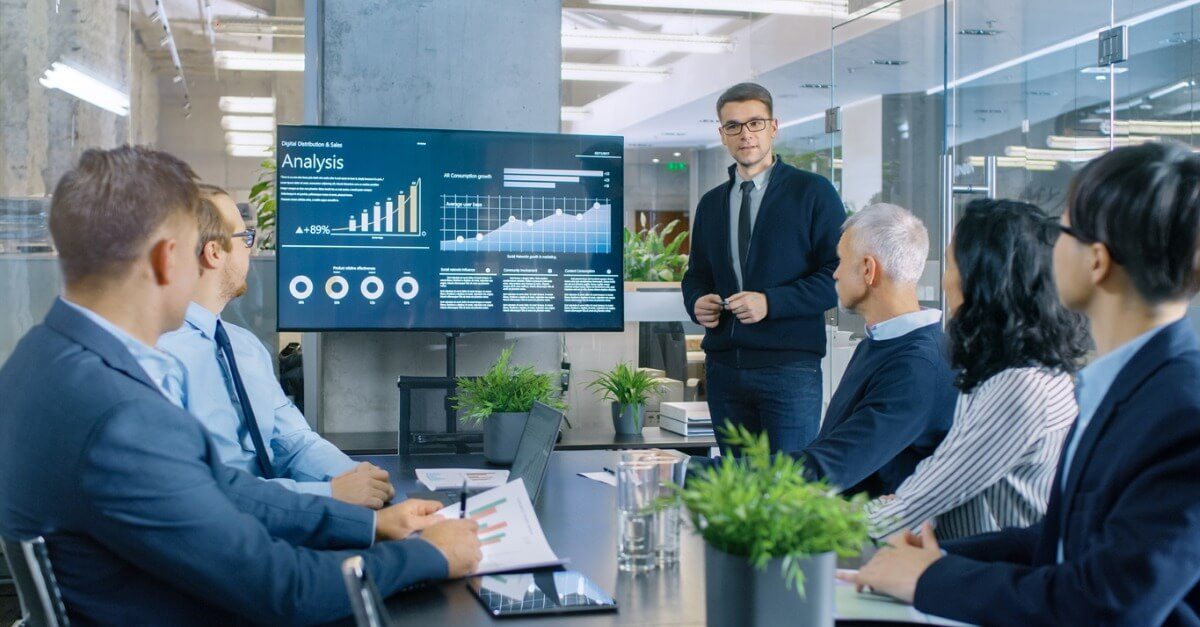
What is the Self-Service Analytics approach?
We’ve covered what the meaning of self-service analytics is and the key steps to prepare for self-service analytics in previous deep dive articles, but for those readers unfamiliar with the term, essentially think of it as a modern approach to business intelligence and analytics that focuses on tools that democratize data for everyone, not just those with technical know-how.
How did the Self-Service Analytics approach start?
The paradigm shift toward empowering individuals to explore and analyze data began with a pivotal change in thinking towards the role of BI and analytics in the organization.
The process of IT building dashboards or reports for a user often relies too heavily on the expectation or assumption the user would understand what the data presented to them is saying or that they would know what to do next after a provided explanation from IT.
The centralized BI model for information needs beyond daily functional and operational necessities (compliance, sales tracking, etc) is simply not sufficient in most organizational use-cases to guarantee a business professional will truly value or engage with the data and insights they request from IT.
But letting users freely explore data also came with challenges: How can untrained professionals leverage such advanced analysis tools on their own, when they need insights? How can they navigate the complexities of data transformation, preparation, or querying without training? This required analytics vendors to rebuild and re-shape their tools to become consumer-focused, rather than analyst-focused. This movement is referred to as the augmented consumer.
With BI solutions becoming increasingly sophisticated through the integration of augmented analytics technologies such as automation, artificial intelligence (AI), machine learning (ML) and natural language query (NLQ), vendors recognized they could leverage these powerful tools to streamline the process of analysis and open up BI tools to more people, becoming consumers driven by data rather than restricted by its complexity.
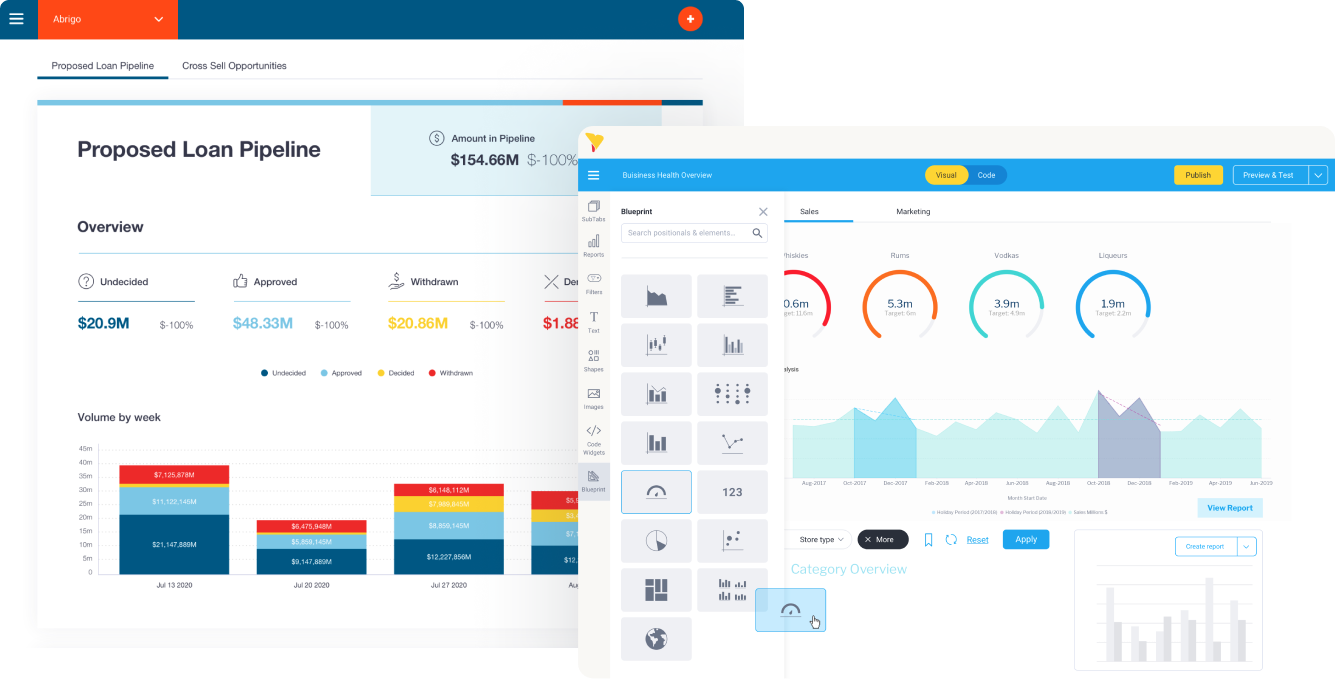
What are the Pros and Cons of Self-Service Analytics?
Self-service analytics brings an immense amount of advantages to the average organization, which we’ve covered in more complete detail in our article, Self-Service Analytics: 5 Key Benefits, and comparatively few disadvantages.
One major consideration for businesses adopting a self-service BI tool is to be sure you have the appropriate buy-in (i.e. data culture established) from users, and that requires proper education of the value and capability such a tool will bring to their daily business workflows. Otherwise, your shiny new BI solution may go underutilized, or even misused.
Yellowfin is centered around providing a self-service BI experience for all users. The platform leverages sophisticated AI, automation, machine learning and natural language technologies to empower data experts and everyday business professionals to create, consume and share data insights, helping open up data-driven analysis and decision-making across the full organization.
Yellowfin achieves its self-service BI ideal by offering three pivotal analysis features, each tailored toward users at different levels and needs of their own analytics journey:
1. For business users (consumers): Guided Natural Language Query (Guided NLQ)
2. For intermediate analytics users (explorers): Assisted Insights and Quick Charts
3. For data analysts (experts): Advanced Report Builder and Yellowfin Signals
All of these features are unique to the Yellowfin BI suite of tools, and continuously updated and refined to provide the powerful outcomes augmented consumers can achieve with self-service analytics right now.
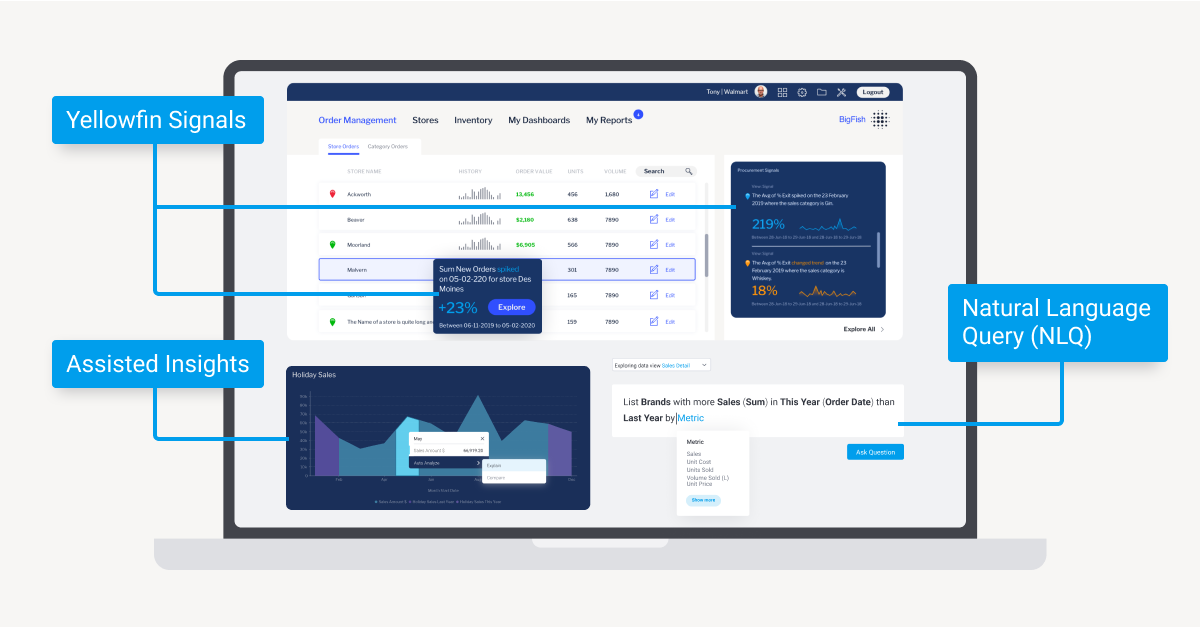
Self-Service Analytics: Explore Yellowfin
Learn how Yellowfin can provide efficient, streamlined, self service analytics for every type of business user, regardless of skill level - try our demo today.