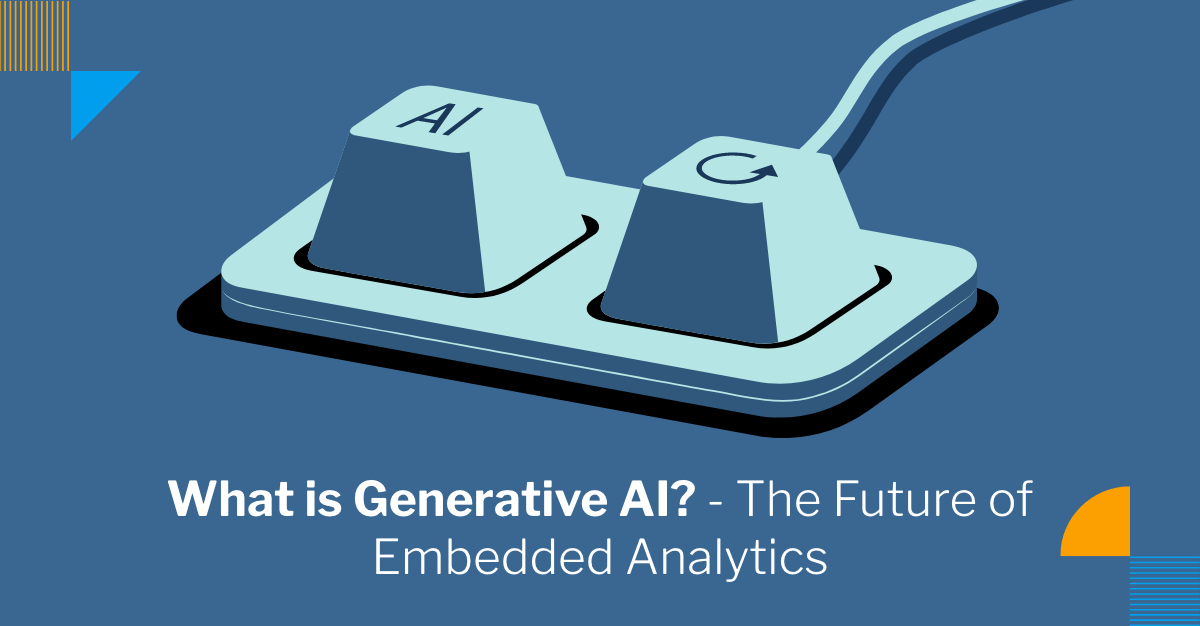
What is Generative AI? - The Future of Embedded Analytics in 2025
The world of business intelligence (BI) and analytics is rapidly evolved from descriptive to predictive, and now, to generative.
Generative AI, a cutting-edge technology, is making its mark in embedded analytics solutions. These powerful AI analytics tools are assisting business users in analyzing their data sources more quickly and easily, generating new data sets for deeper insights, and helping make the embedded BI experience more accessible for more people.
With generative AI, embedded analytics tools are significantly transforming decision-making processes across several industries. But the journey is not without challenges - data privacy, computational requirements, and the need for skilled professionals are just some of the hurdles you need to address to leverage AI analytics effectively.
This article explores the integration of generative AI for data analytics. It delves into its current state, future potential, and the challenges it presents in 2025 and beyond.
What is generative AI?
Generative AI is a branch of artificial intelligence (AI) that enables individuals and businesses to produce original content, data, and solutions based on user input.
Unlike conventional AI systems that focus on analyzing and interpreting existing data for decision-making and predictions, generative AI employs sophisticated algorithms to generate novel outputs that encompass a wide spectrum, from text and images (as seen in popular consumer tools like ChatGPT and Google Gemini) all the way to intricate data models, data visualizations and simulations, which are seen in certain data analytics tools, such as Yellowfin BI.
Generative AI differentiates itself from other traditional analytics technologies by combining the use of automation, machine learning (ML), large language models (LLMs), and natural language technologies (NLT) such as natural language processing (NLP) and natural language query (NLQ) to tailor outcomes based on unique user inputs and behaviors, helping ensure that every generated output is as accurate and relevant as possible so the user of the generative analytics tool can make informed decisions.
Related reading: Deciphering the Hype and Reality of ChatGPT for BI and Analytics
Generative AI for data analytics explained
Within the context of business intelligence and analytics software solutions, generative AI falls under the banner of augmented analytics (also known as AI analytics), a concept that describes the use of multiple AI technologies (including generative AI) to transform how our data analytics solutions can be built, consumed and shared.
The defining theme of augmented analytics is the objective of using modern AI software and technology to help democratize access to data and streamline the usage of analysis tools for everyone, from line-of-business professionals to developers to data analysts.
Instead of having to manually dissect data, or consult technical experts and data scientists to have insights explained, generative AI for data analytics can be deployed to generate insights and these inputs, saving users time and effort while providing a true self-service analytics user experience. This can be a huge benefit for enterprise and independent software vendors (ISVs) looking to enhance the capabilities and value of their existing analytics solutions. By harnessing vast datasets to identify underlying patterns with the right generative AI analytics tools, your users can:
- Find helpful, actionable insights faster (using AI to sift through vast data)
- Compare and analyze data-sets easier (using AI to generate analysis)
- Ask the right questions to find relevant answers (using AI to generate question parameters and suggestions)
The adoption of generative AI is poised to increase significantly in the coming years, according to a forecast by Gartner, with 30% of enterprises expected to have integrated AI-augmented strategies (including augmented analytics) into their development and testing processes by 2025, an increase of 25% from Gartner's earlier 2021 study.
The growing adoption and importance of generative AI as part of the analytics tools we use to analyze data is also creating a new class of analyst that isn't necessarily a technical expert, called the augmented consumer - and it's important you brush up on the concept.
Read more: 3 Key Drivers and Considerations for AI Analytics in 2025
Generative AI and embedded analytics: How does it work?
Embedded analytics is a form of data analytics solution that integrates analytical capabilities directly into business applications, bringing the data analysis process directly into your workflows instead of being a separately accessed tool. Generative AI synergizes well with embedded analytics solutions, as it is an effective and fast way to bring these sophisticated tools into the users' everyday analytics experience.
Generative AI operates within embedded analytics by utilizing advanced algorithms to analyze existing data and generate new insights that were previously unattainable. This process involves training data and large language models on large volumes of historical data, allowing the AI to understand patterns and relationships within it. Once trained, these machine learning models can generate predictions, compare and simulate scenarios, and even create best practice examples using the embedded analytics platform's existing visualization and reporting modules.
When deployed correctly, generative AI and other AI-powered technologies can help make the existing analytical experience more accessible, and also enable your organization and/or customers to explore "what-if" scenarios, facilitating more informed decision-making and an ability to move beyond traditional reporting and analysis, to embrace a more proactive approach to data utilization and data exploration.
Learn more: The Ultimate Guide to Embedded Analytics: A Starter Kit for Embedded BI
What is the best generative AI tool for data analysis?
Today, some embedded analytics vendors (such as Yellowfin) deploy generative AI as part of their many varied capabilities to help further streamline the process of data preparation, insight discovery, and data-driven business decisions, and empower more analytics users to become augmented consumers.
Embedded analytics tools that provide generative AI capability not only offers your end-users valuable historical context to their data-sets, but also helps them forecast future scenarios, automate data discovery and streamline data querying.
Yellowfin specifically incorporates sophisticated AI analytics features into its business intelligence suite, making it a powerful tool for both enterprises and ISVs looking to utilize AI analytics. Here's a concise summary of Yellowfin's AI-specific functionalities, along with links to more comprehensive resources:
Accessible AI: Yellowfin lowers the entry barrier for users with limited data analysis expertise, with its natural language-powered AI NLQ feature that uses AI to allow any analytics user to ask a question of their data in free text using natural language (no jargon) and have the system structure the question with the proper syntax automatically, and instantly generate the relevant, accurate answers they are looking for. You can see AI NLQ in action in the video below, or read more about it in our explainer guide, What is AI NLQ?
Automated analytics: Yellowfin's gen AI algorithms are capable of autonomously identifying and conveying significant patterns and anomalies in data with its Signals feature, simplifying intricate data analysis tasks.
Predictive insights: By harnessing machine learning and natural language, Yellowfin can forecast trends and behaviors, and elucidate or compare the importance or specific data-sets to end-users with its Assisted Insights feature, empowering more people to access insights and utilize data for proactive decisions. Check it out in the demo video below.
Real-world applications of generative AI analytics
Generative AI isn't just a tool; it's a driver of innovation and growth. Across various sectors, it is unlocking new potential and transforming how businesses operate. Its influence will only continue to grow as more industries recognize its value. Here are a few real-world examples:
1. AI-powered diagnostics
Some analytics platforms in healthcare now incorporate generative AI models. These models can analyze medical images like X-rays, MRIs, and CT scans, assisting radiologists in identifying potential abnormalities. For instance, a platform might use AI to generate reports summarizing key findings in medical images, highlighting potential areas of concern for further investigation.
2. Better market insights
Finance companies are adopting analytics platforms leveraging generative AI to provide deeper market insights. Such platforms can help finance businesses generate concise and insightful reports on market trends, competitive advantage landscapes, and potential investment opportunities. For example, an AI model might analyze vast amounts of financial news, social media sentiment, and market data to generate a report summarizing the key factors influencing the performance of a particular stock or sector.
3. AI-powered customer segmentation
Many marketing analytics platforms now offer generative AI features to segment customers based on their behaviors, preferences, and demographics with greater granularity. For example, an AI model might analyze customer data to identify distinct customer segments with unique needs and preferences. This allows marketers to tailor their marketing campaigns more effectively to specific customer groups, improving campaign ROI.
Retailers are also using analytics solutions with generative AI to optimize supply chains and enhance customer engagement. Using gen AI tools to analyze purchasing behavior, retailers can better predict trends and adjust marketing strategies accordingly, leading to increased customer satisfaction and loyalty.
4. Enhanced IoT and edge computing
Generative AI boosts Internet of Things (IoT) efficiency by processing data locally through edge computing. IoT devices generate vast amounts of data in real-time. With embedded AI, these devices can analyze data at the source. This reduces latency and leads to quicker decision-making. In industries like manufacturing, this capability is particularly invaluable, as companies can better optimize operations and reduce downtime with faster insights and actions.
5. Predictive maintenance and anomaly detection
Generative AI excels in predictive maintenance use cases for industries such as manufacturing by helping organizations foresee equipment failures as part of their regular data analysis processes. It analyzes patterns to anticipate issues before they arise, to proactively minimize downtime and save on costs.
Top generative AI challenges in data analytics
It's undeniable that investing in embedded analytics solutions that leverage generative AI offers significant advantages. However, successfully realizing these benefits necessitates careful consideration and proactive measures to address the inherent challenges associated with adopting generative AI tools as part of your analytics investment.
1. Data privacy
The use of sensitive data within generative AI models carries the real risk of data breaches, potentially exposing confidential information. One of the most important things to look out for when selecting an embedded analytics solution offering generative AI tools is that they adhere to stringent data privacy regulations like GDPR and CCPA, ensuring compliance and minimizing the risk of legal repercussions.
Implementing data minimization techniques as part of your business processes is crucial. Only necessary data should be used for training AI models, reducing the attack surface and minimizing the potential impact of a breach. Additionally, exploring differential privacy techniques, or partnering with a BI vendor that can guide you in this area, can help protect individual user data while still enabling valuable insights.
Ultimately, building trust with your users is paramount. Be transparent about how user data is collected, used, and protected. Clearly communicating data privacy practices and obtaining explicit user consent will foster trust and encourage adoption among your internal user-base and customers of your newly invested generative AI analytics solution.
2. Data quality
The quality of insights generated by AI models is directly dependent on the quality of the input data. Inaccurate, incomplete, or biased data will inevitably lead to flawed and misleading results. As such, your business needs to be prepared to conduct robust data cleaning and preprocessing, including identifying and handling missing values, detecting and correcting inconsistencies, and addressing potential biases in the data.
Continuous monitoring of data quality is also crucial. Implementing mechanisms to identify and address data quality issues proactively will ensure the ongoing accuracy and reliability of your newly invested AI-powered analytics tools. If you require assistance in establishing a framework, we recommend selecting BI product vendors who provide built-in data preparation suites to ensure data quality is up-to-standard.
3. Computational requirements
Training and deploying large-scale generative AI models can be computationally expensive. Carefully consider computational resources so you can allocate resources effectively. Leveraging analytics solutions combined with cloud computing platforms provides access to scalable and on-demand computational resources, enabling your business to more efficiently train and deploy AI models.
4. Data governance
Every organization that utilizes AI as part of data collection and analysis processes should establish clear data governance frameworks that prioritize transparency and compliance. This involves creating policies that dictate how data is collected, stored, and utilized, ensuring that all stakeholders understand their roles in maintaining data integrity, especially when the use of AI to create models and simulations based on your sensitive historical data is concerned.
Preparing for future of generative AI and embedded analytics
The generative AI revolution in analytics solutions, particularly embedded analytics, is not just on the horizon - it is already here. Understanding and adapting to these AI-driven solutions early can lead to transformative changes, and help you stay competitive and provide value to your analytics users.
To help you get started on your journey, Yellowfin has a multitude of free content resources unique to help you get up-to-speed on what generative AI offers, and the broader technology umbrella of augmented analytics (also called AI analytics) and what it can bring to your customers and business. We recommend starting with our free whitepaper download: Embedding AI-Powered Analytics Into Your Application.
Next steps: Explore Yellowfin generative AI embedded analytics
Yellowfin is a feature-rich business intelligence and analytics platform with several generative AI analytics tools ready for you to leverage to enhance your users' analysis experience. Learn more by speaking to our team today.